PFENet++: Boosting Few-shot Semantic Segmentation with the Noise-filtered Context-aware Prior Mask
First Author: Xiaoliu Luo
Introduction:
In recent years, the rapid advancement of computer computing capabilities and the availability of large-scale annotated data have propelled the swift development of artificial intelligence technologies, particularly those rooted in deep learning. Nevertheless, in numerous real-world application scenarios, the acquisition of samples poses a significant challenge, compounded by the exceedingly difficult task of annotating them. The focus has shifted towards the pivotal question of how to effectively learn from a limited number of samples.
This paper revisits the prior mask guidance proposed in “Prior Guided Feature Enrichment Network for Few-Shot Segmentation (PFENet)”. The prior mask serves as an indicator that highlights the region of interests of unseen categories, and it is effective in achieving better performance on different frameworks of recent studies. However, the current method directly takes the maximum element-to-element correspondence between the query and support features to indicate the probability of belonging to the target class, thus the broader contextual information is seldom exploited during the prior mask generation. To address this issue, a novel framework named PFENet++ is proposed, which boosts few-shot semantic segmentation with the noise-filtered context-aware prior mask. Specifically, PFENet++ first proposes the Context-aware Prior Mask (CAPM) that employs region matching to generate multi-scale context-aware prior masks for better locating the objects in query image. Moreover, PFENet++ further incorporates a lightweight Noise Suppression Module (NSM) to screen out the unnecessary responses, yielding high-quality masks for providing the prior knowledge. Both two contributions are experimentally shown to have substantial practical merit, and PFENet++ significantly outperforms the baseline PFENet as well as all other competitors on three challenging benchmarks PASCAL-5i, COCO-20i and FSS-1000. The new state-of-the-art performance is achieved without compromising the efficiency, manifesting the potential for being a new strong baseline in few-shot semantic segmentation.
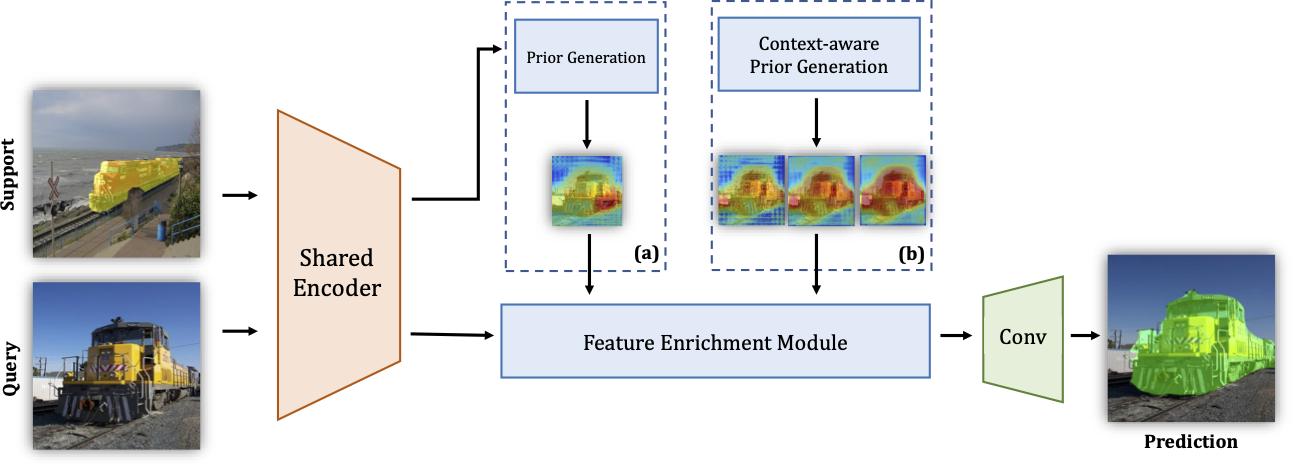
Figure 1: The difference between PFENet and PFENet++ lies in their prior generation methods depicted in dashed boxes (a) and (b) respectively. The original prior generation method (a) only takes the maximum values from the one-to-one correspondence, while our new pipeline (b) yields preferable prior masks by decently incorporating additional contextual information with the proposed two sub-modules, i.e., CAPM and NSM. Besides, (a) only leverages the high-level features to yield prior masks, while (b) makes use of both high- and middle-level features. Adding middle-level features to (a) does not boost its performance.
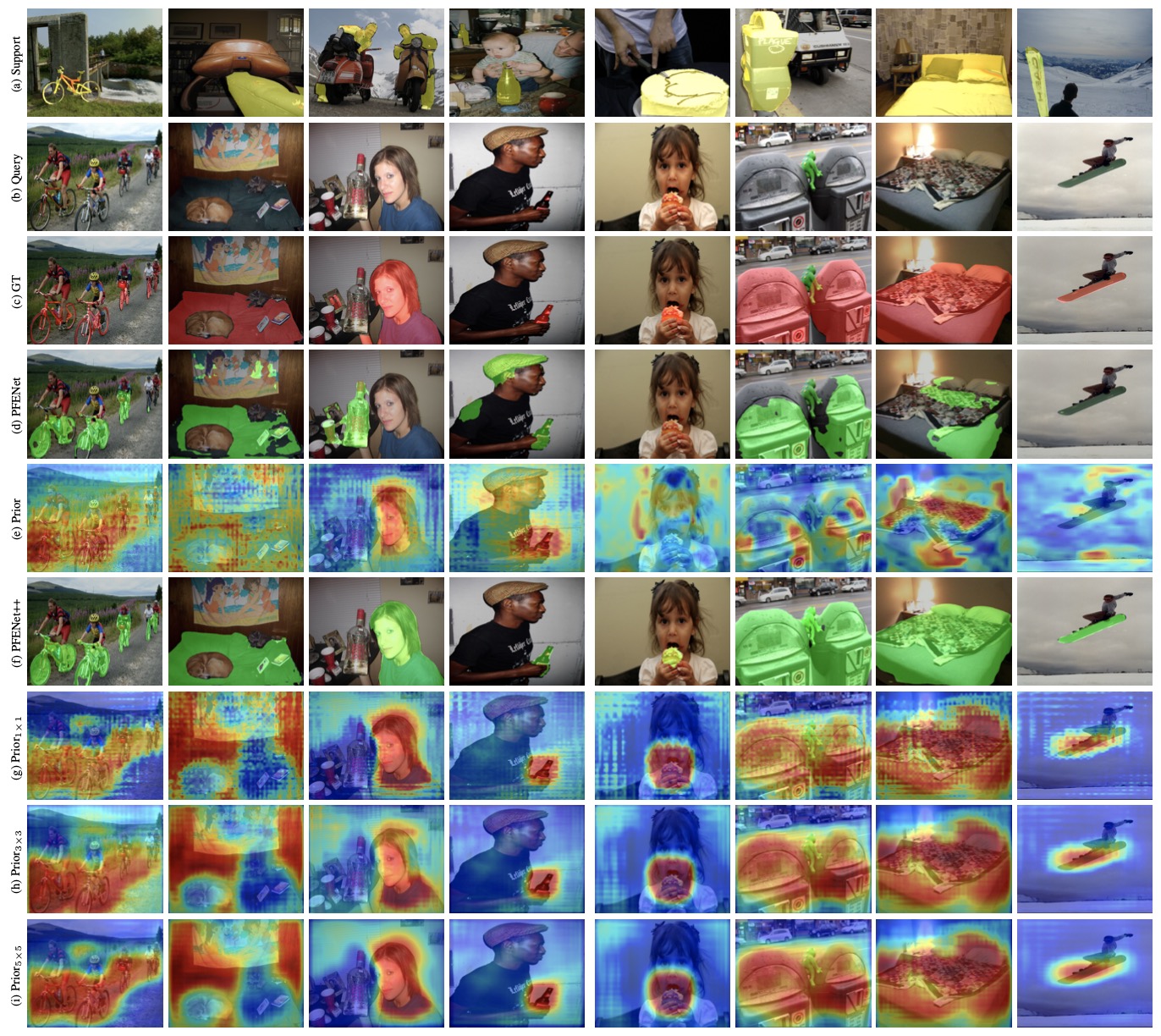
Figure 2: Qualitative results of the proposed PFENet++ and the original PFENet. The right samples are from COCO and the left ones are from PASCAL-5i. From top to bottom: (a) support images; (b) query images; (c) ground truth of query images; (d) predictions of PFENet; (e) the vanilla prior mask of PFENet; (f) the predictions yielded by 1 × 1, 3 × 3, 5 × 5 patches, respectively.